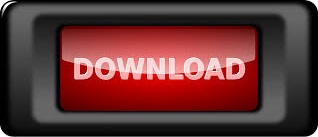
If compute_target and type(compute_target) is AmlCompute: Vm_size = os.environ.get("AML_COMPUTE_CLUSTER_SKU", "STANDARD_D2_V2")Ĭompute_target = ws.compute_targets For using GPU VM, set SKU to STANDARD_NC6 from import AmlComputeįrom import ComputeTargetĬompute_name = os.environ.get("AML_COMPUTE_CLUSTER_NAME", "cpu-cluster")Ĭompute_min_nodes = os.environ.get("AML_COMPUTE_CLUSTER_MIN_NODES", 0)Ĭompute_max_nodes = os.environ.get("AML_COMPUTE_CLUSTER_MAX_NODES", 4)
#JUPYTER NOTEBOOK TUTORIAL CODE#
If you created a compute cluster in the quickstart, make sure compute_name in the code below uses the same name. If the compute resource is already in the workspace, the code uses it and skips the creation process. It sets up a cluster that will scale down to 0 when not in use, and can scale up to a maximum of 4 nodes.Ĭreation of the compute target takes about five minutes. The code below creates the compute clusters for you if they don't already exist in your workspace. You will submit Python code to run on this VM later in the tutorial. In this tutorial, you create Azure Machine Learning Compute as your training environment.

A workspace can have multiple experiments: from re import ExperimentĮxperiment_name = 'Tutorial-sklearn-mnist'Įxp = Experiment(workspace=ws, name=experiment_name)Ĭreate or attach an existing compute targetīy using Azure Machine Learning Compute, a managed service, data scientists can train machine learning models on clusters of Azure virtual machines. Create an experimentĬreate an experiment to track the runs in your workspace. You may be asked to authenticate to your workspace the first time you run the following code. Print(ws.name, ws.location, ws.resource_group, sep='\t') # load workspace configuration from the config.json file in the current folder. If you run the code elsewhere, you'll need to create the file. The compute instance has a copy of this file saved in its root directory. om_config() reads the file config.json and loads the details into an object named ws.
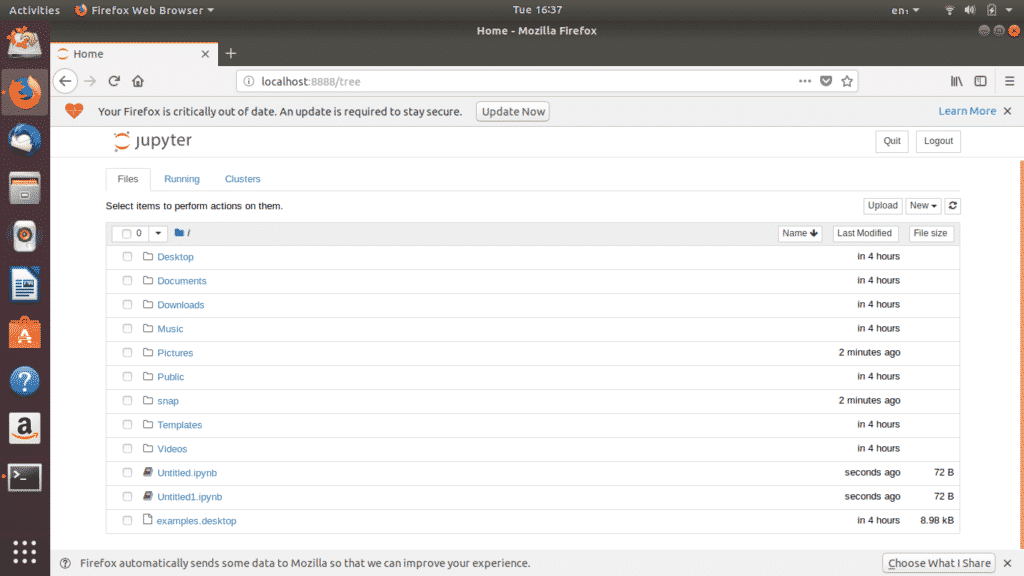
Print("Azure ML SDK Version: ", )Ĭreate a workspace object from the existing workspace. Also display the Azure Machine Learning SDK version: %matplotlib inline Import Python packages you need in this session. Create a remote compute target to use for training.Create an experiment to track all your runs.Connect to a workspace, so that your local computer can communicate with remote resources.Set up your development environmentĪll the setup for your development work can be accomplished in a Python notebook. Or, run the entire notebook by choosing Run all from the top toolbar. To run a single code cell in a notebook, click the code cell and hit Shift+Enter. Switch to the Jupyter Notebook now if you want to read along as you run the code. The rest of this article contains the same content as you see in the notebook. Open the tutorials folder that was cloned into your User files section. Select your folder to clone the tutorials folder there. button at the right of the tutorials folder, and then select Clone.Ī list of folders shows each user who accesses the workspace. This number represents the current release for the Python SDK. Open the folder with a version number on it.


Select your subscription and the workspace you created. Sign in to Azure Machine Learning studio. This consolidated interface includes machine learning tools to perform data science scenarios for data science practitioners of all skill levels. You complete the following experiment setup and run steps in Azure Machine Learning studio. For this tutorial, once you've cloned the tutorials folder, open the img-classification-part1-training.ipynb file from your tutorials/image-classification-mnist-data folder. The video helps you understand the process, but shows opening a different file.
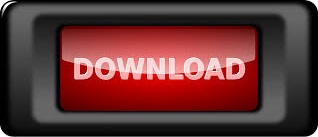